Presenters
Prof. Anthony Bagnall
University of Southampton, United Kingdom
Tony is a Professor in Computer Science at the University of Southampton. His primary research interest is in developing algorithms for time series machine learning, including classification, regression and clustering, and finding novel areas of application. He is currently PI on a three year EPSRC grant called aeon: a toolkit for machine learning with time series that is supporting this tutorial. He has ongoing research collaborations with scientists on four continents and a track record of consultancy with industrial partners. He is an action editor for ECML 2024, is on the editorial board for the Data Mining and Knowledge Discovery journal and regularly reviews for data mining conferences such as KDD, ICDM and SDM. He helps maintain the time series classification archive that has been used in thousands of publications.
Dr. Matthew Middlehurst
University of Southampton, United Kingdom
Matthew is a senior postdoctoral researcher on the ESPRC aeon grant and is a core developer for the aeon toolkit. His research interests are time series classification and regression, with a keen interest in developing open source software for the field and promoting reproducibility. His group has developed the HIVE-COTE family algorithms for time series classification, which have a good claim to represent state-of-the-art in the field.
Prof. Germain Forestier
Université de Haute-Alsace, France
Germain is a university professor at the University of Haute-Alsace in Mulhouse France. He is part of the IRIMAS research institute, for which he is head of the computer science department. His research is mainly oriented around time series analysis, with a speciality in deep learning models. He is co-author of the deep learning review for time series classification for which the tutorial will be based on mostly, as well as new papers which he is co-authored as well. His contributions to the deep learning community in time series classification have been significant recently.
Ali Ismail-Fawaz
Université de Haute-Alsace, France
Ali is a PhD student supervised by Germain Forestier, Jonathan Weber, Maxime Devanne and Stefano Berretti, stationed at the IRIMAS institute of the University of Haute-Alsace in France. He is a core developer for the aeon toolkit and is leading the deep learning part of the package with a recent kick off for a deep clustering module. His main research topic is focused on time series analysis, with a speciality in deep learning. His work has addressed different tasks such as classification and clustering.
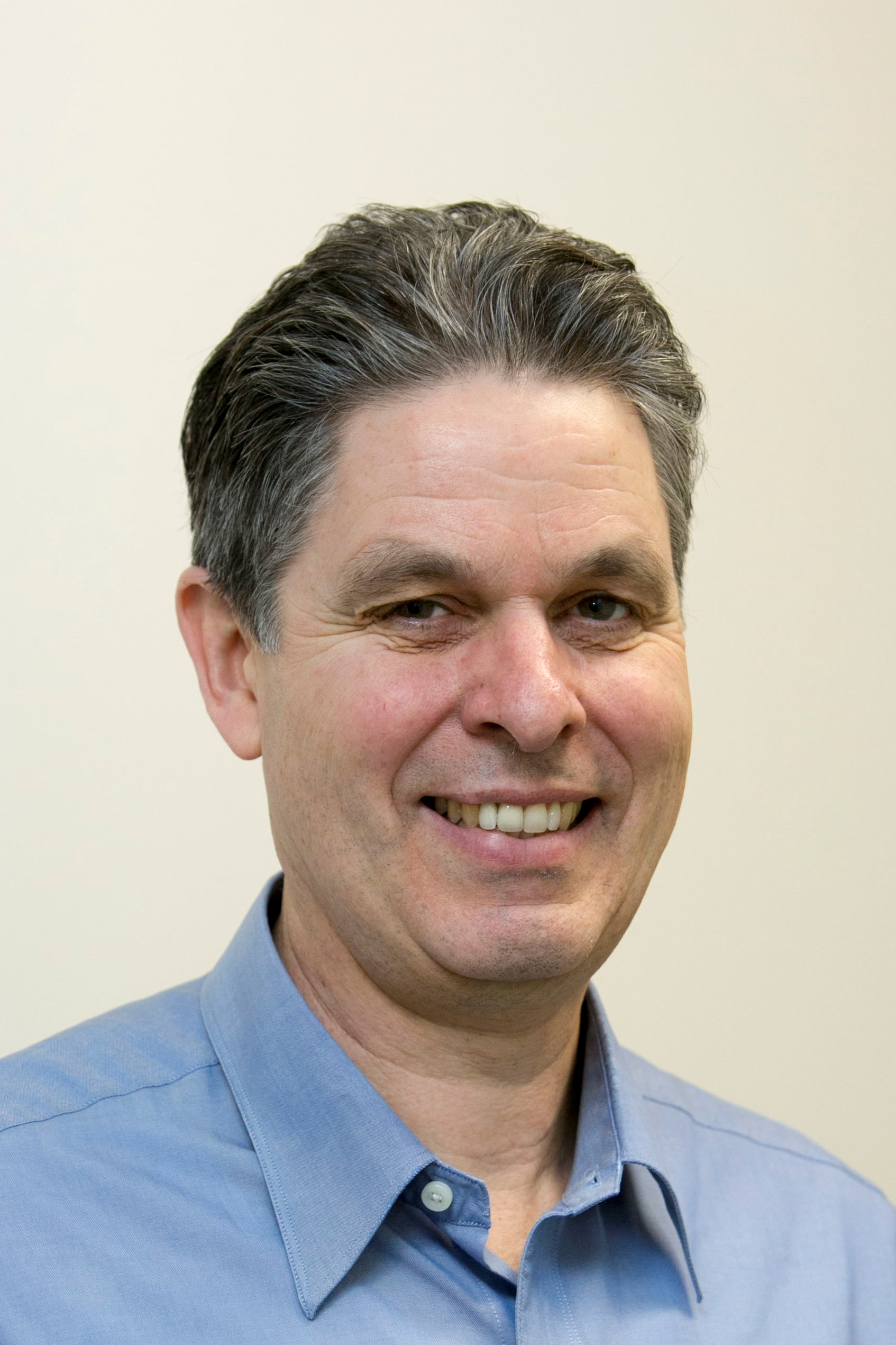
Prof. Geoffrey I. Webb
Monash University, Australia
Geoff is an eminent and highly-cited data scientist. He was editor in chief of the Data Mining and Knowledge Discovery journal, from 2005 to 2014. He has been Program Committee Chair of both ACM SIGKDD and IEEE ICDM, as well as General Chair of ICDM and member of the ACM SIGKDD Executive. He is a Technical Advisor to machine learning as a service startup BigML Inc and to recommender systems startup FROOMLE. He has developed many useful machine learning algorithms that are widely deployed. His many awards include IEEE Fellow, the inaugural Eureka Prize for Excellence in Data Science (2017), the Pacific-Asia Conference on Knowledge Discovery and Data Mining Distinguished Research Contributions Award (2022) and the IEEE International Conference on Data Mining 10-year Highest Impact Award (2023). He has thrice been recognised by The Australian Research Magazine as Australia’s leading Bioinformatics and Computational Biology researcher.
Contributors
Dr. Antoine Guillaume
Novahé and Constellation, France
Antoine is a R&D manager at Novahé and Constellation. He is currently studying explainability, uncertainty quantification and carbon footprint reduction in the context of ML products. He specializes in time series machine learning and similarity search with an emphasis on rare event prediction, and works on applications in industry 4.0 and IT systems.
Dr. David Guijo-Rubio
University of Alcalá, Spain
David is a postdoctoral researcher at the University of Alcal ́a, Spain. He is working on time series extrinsic regression and clustering, and is a core developer for the aeon toolkit. His research interests are time series machine learning, explainability, evolutionary artificial neural networks, and ordinal classification. He is also interested in the applications of such techniques to real-world problems related to biomedicine and renewable energies.
Dr. Chang Wei Tan
Monash University, Australia
Chang Wei is a research fellow at Monash University, who specializes in time series analysis and machine learning applications. His PhD thesis led to significant advancements in Scalable Time Series Classification, earning him the prestigious Mollie Holman medal. His innovative algorithm for Dynamic Time Warping (DTW) parameter learning earned him a best paper award at the SDM 2018 conference. Chang Wei also applies his knowledge to real-world problems, such as improving railway track maintenance with the Institute of Railway Technology. His current research continues to focus on scalable time series classification, Time Series Extrinsic Regression (TSER) and the application of EEG devices for medical diagnosis and driver distraction detection. In addition to his research, Chang Wei also lead a project on Computational Cultural Understanding with DARPA and contributes to autonomous supply chain demand forecasting solutions for Stemly, a supply chain company.
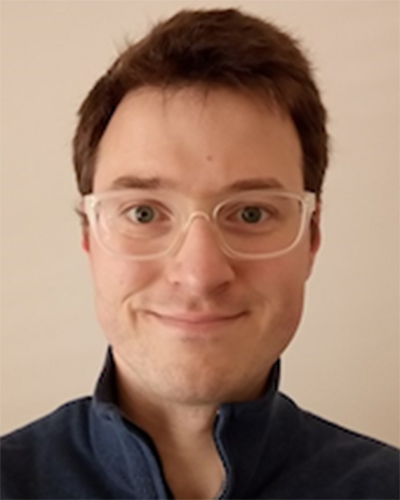
Dr. Angus Demptster
Monash University, Australia
Angus is a research fellow at Monash University, where he also received his PhD. His research interests include scalable methods for time series classification, benchmarking and performance evaluation, and fundamental methods for machine learning.